Can I get a loan? How much is my insurance premium? Am I eligible for social security benefits? Today, many important decisions are made by algorithms that analyze our personal data. These systems are often highly efficient—but are they fair?
Four short films held in a charming retro aesthetics explain how algorithms influence our lives. (© Tristesse)
Algorithms are increasingly being used to make fundamental decisions in our lives: Will I get a loan? How high is my insurance premium? Am I entitled to welfare benefits?
These systems are often highly efficient. They have been trained with data sets from many people and, as a result, have learned to recognize patterns in these data sets. Those patterns are used, for example, to look for indications of the degree of risk that a particular individual will not repay a loan. In this context, it is important not only that the algorithms work well – in other words, that they do what they are supposed to – but also that decisions made based on them are fair.
Even algorithms that, at first glance, make seemingly less vital decisions have an impact on our lives and how we live together as a society: How do social media algorithms decide which content to show me? Which ads do I see in my browser? Is Google Maps directing drivers through my neighborhood because of a construction site? Which bus stops does the algorithm suggest for the new transport network? Does it consider the need for connections to the local post office as well as fast links to the city center?
The question is whether these systems are fair and reduce social disparities or whether, on the contrary, they maintain or even reinforce such inequality. That is not a purely technical issue, as algorithms are programmed by human beings. As a result, opinions and social prejudices always filter through into the algorithms – often quite unintentionally. In recent years, cases of discrimination due to algorithm-based decision-making systems have repeatedly come to light.
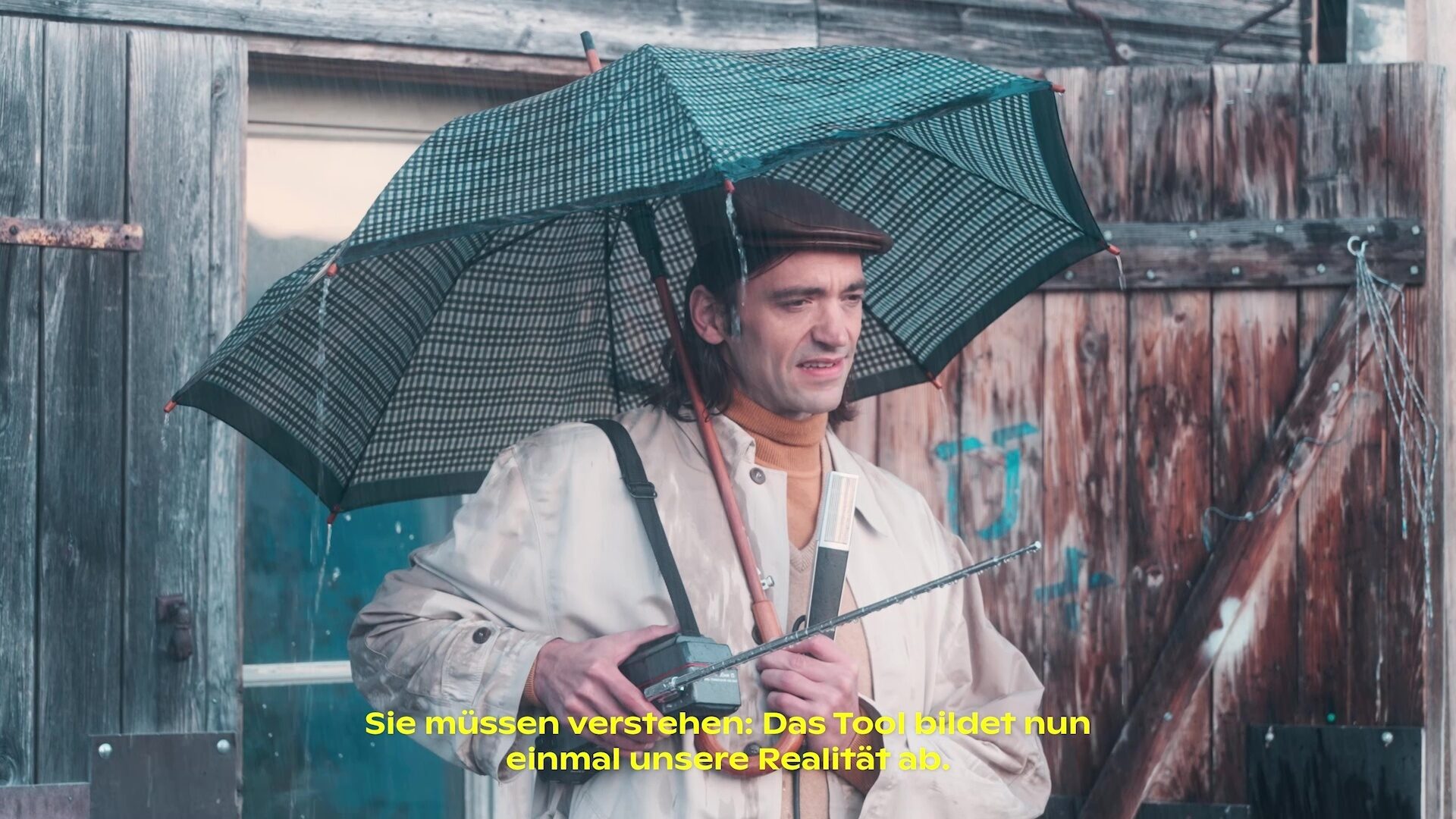
Algorithm-based hiring, unemployment, credit scores or child welfare - the topics of the films are very diverse. (© Tristesse)
In the exhibition, four films are screened that draw on real events which could readily be imagined unfolding in many countries. They show the various aspects of inequality that need to be discussed when using algorithm-based decision-making systems.
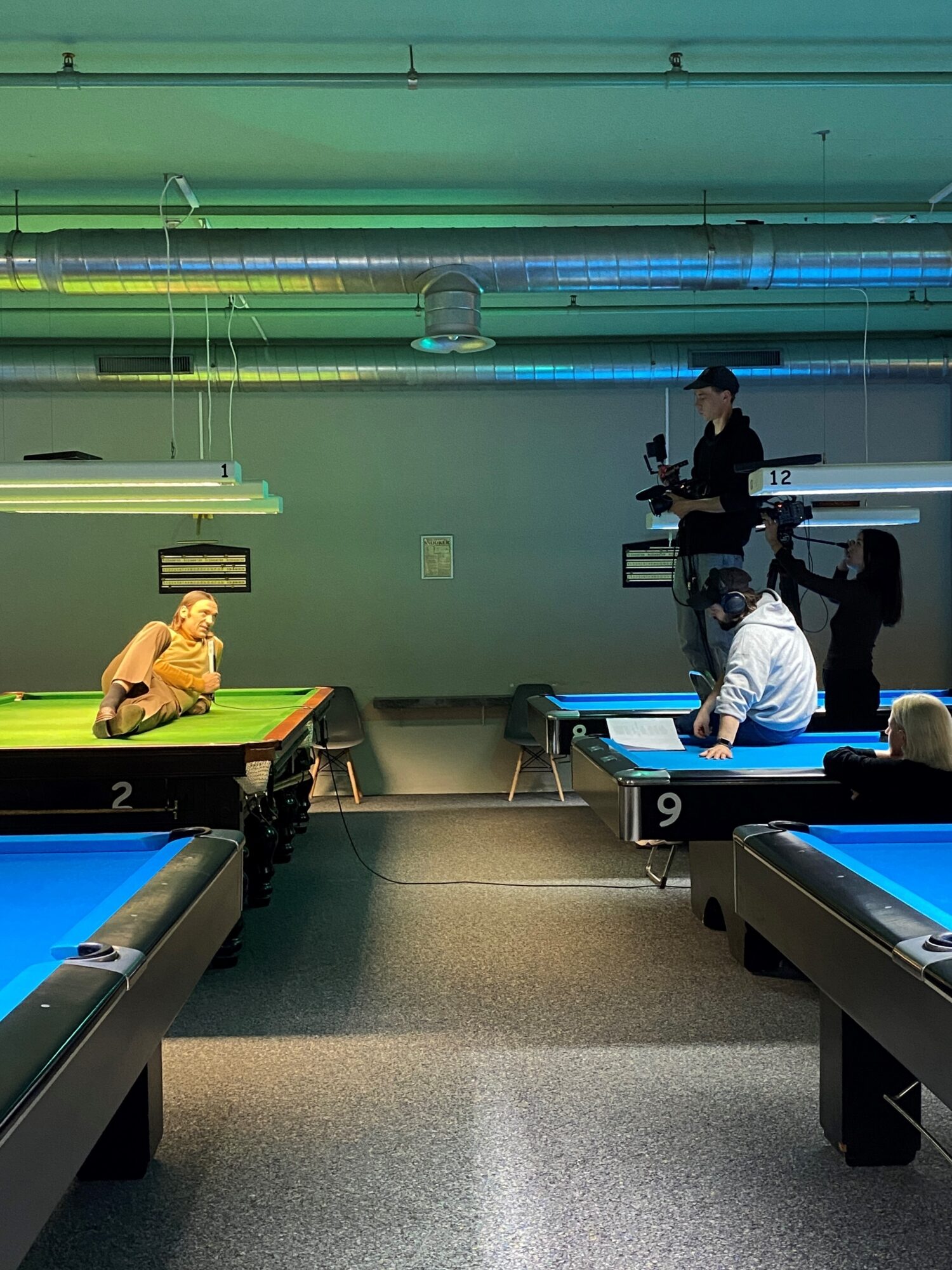
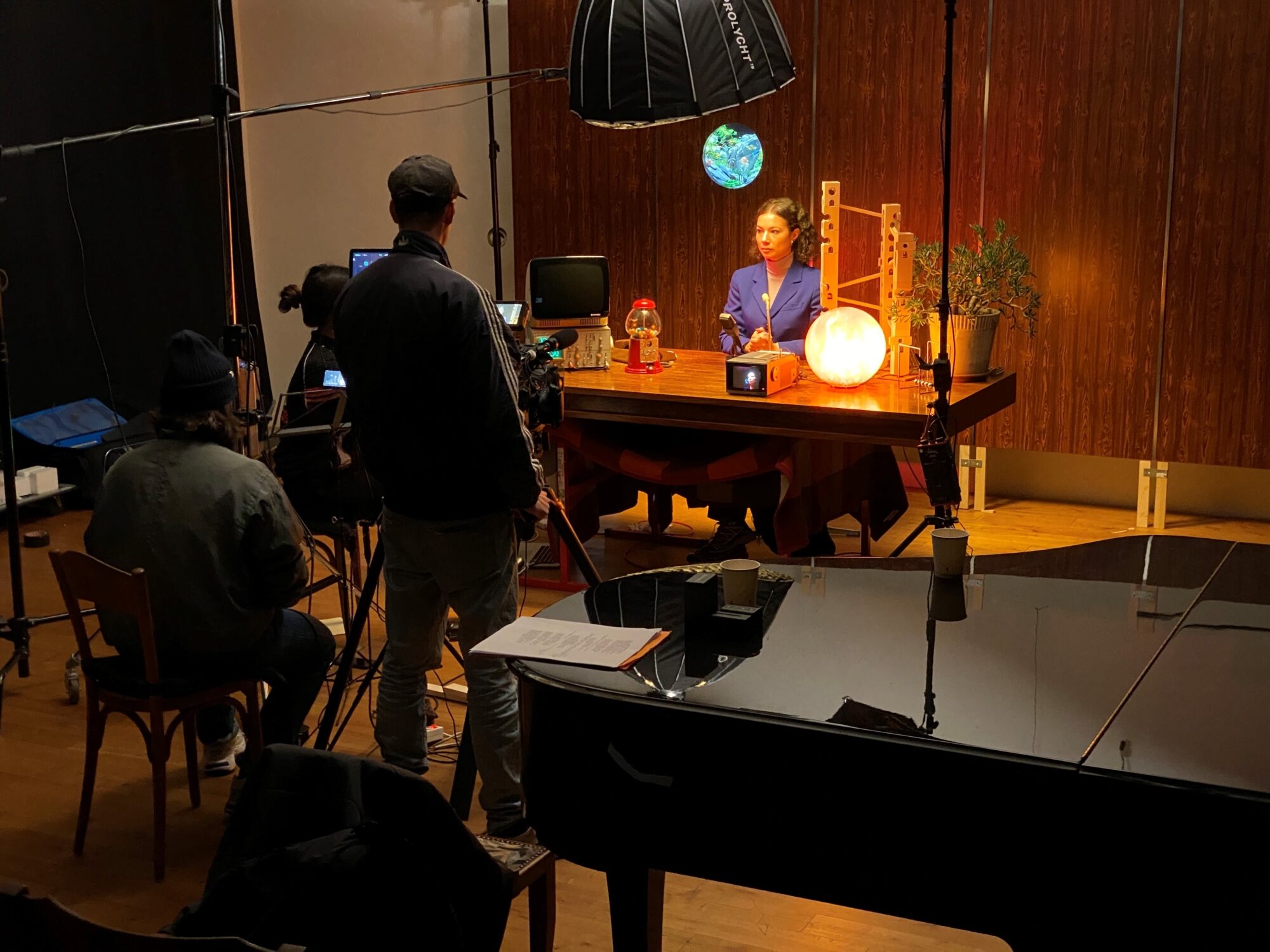
Scenes from the shoot. (© Tristesse)
Further information on real-world applications provides an overview of where and how algorithms are utilized in the private and public sectors. Is the use of algorithms in these examples equitable? Does it make sense? Are there cases in which it is too risky? And how could negative effects be mitigated?
These questions are also addressed by several research projects at the Zurich University of Applied Sciences (ZHAW) and the organization AlgorithmWatch Switzerland, which jointly developed this exhibit.
The Institute for Data Analysis and Process Design (Zurich University of Applied Sciences, School of Engineering) develops algorithms for data-based decision making for a wide variety of application contexts. For several years, the researchers have investigated the social implications of such algorithms, in particular the question of their social justice (“algorithmic fairness”): What exactly does “fair” mean in a given context? How can the fairness of algorithms be measured? And how can developers ensure that algorithms are indeed fair?
In an interdisciplinary team of computer scientists, philosophers and economists, the researchers are looking for solutions on how to develop algorithms such that they are not only efficient, but also socially just. The cooperation with the University of Zurich and University of St. Gallen is supported by the Swiss National Science Foundation and Innosuisse.
AlgorithmWatch Switzerland (https://algorithmwatch.ch) is a non-profit research and advocacy organization committed to evaluating and shedding light on algorithmic decision-making processes that have social relevance, meaning they are used either to predict or prescribe human action or to make decisions automatically. AlgorithmWatch analyzes the effects of algorithmic decision-making processes on human behavior with journalistic and scientific research and points out ethical conflicts. These effects are explained to the general public. In order to maximize the benefits of algorithmic decision-making processes for society, AlgorithmWatch Switzerland supports the development of more transparent processes — with a mix of technology, regulation and appropriate oversight tools.
We hope to inspire the visitors of this exhibition to also engage in this important discussion.
While the four films exemplify cases of (un)fair algorithms, the posters show real examples from different countries — but this list is not exhaustive. We all interact with such systems — often without realizing it.
Fair or unfair? Each case is different, and depending on the context, the question of fairness and social justice presents itself in different ways. What all cases have in common though is that one can (and should!) always take a close look and ask whether the algorithms treat the affected people fairly or unfairly.
In a county in Pennsylvania, “machine learning” has been used in child welfare protection since 2016. The “Allegheny Family Screening Tool” is designed to help social workers assess the risk of child endangerment when they receive reports of suspected maltreatment. The tool was developed by researchers. It is one of the few examples that places emphasis on transparency in the development process (e.g., through dialogue with stakeholders and the publication of studies on the tool). However, the tool has been criticized by civil society organizations as it could lead to children from poor, Black, and Latinx families being unnecessarily often separated from their parents.
A research group at ETH Zurich and Stanford University has developed a tool to improve the assignment of refugees to resettlement locations within a country. The goal of the improved assignment is to increase the chances of swift integration (measured, for example, as finding work). Empirical results promise a better distribution compared to a random assignment mechanism. The tool is currently being tested in Switzerland, the Netherlands and Canada. The question of whether the new assignment algorithm could lead to discrimination against certain groups of refugees needs to be investigated in detail as part of the practical tests.
From 2014 to 2020, the “System Risk Indication” tool was used in the Netherlands to detect cases of social welfare fraud. The tool analyzes data that is merged from different sources. It is supposed to detect patterns that indicate social welfare fraud and to flag suspicious cases. In 2020, a court banned the use of the tool, arguing that it accessed too much personal data of welfare recipients. The tool has also been heavily criticized as the annual loss of 150 million euros due to welfare fraud is countered by 22 billion euros in tax evasion. In addition, it is unclear how exactly the tool works — this lack of transparency has also caused criticism.
A small town on Sweden's southern coast called Trelleborg is pioneering the digitization of government processes in Sweden. A few years ago, Trelleborg started using a bot for approving welfare applications. The bot was developed in collaboration with an external consulting firm as well as a software company. According to the government agency, the tool saves a lot of time. However, there is resistance to the automation of this process. Journalists and researchers criticize the lack of transparency with which the tool decides on the applications of social welfare recipients.
In order to assess the risk of flight and recidivism of an incarcerated person, the “Fall-Screening-Tool” (“case screening tool”) is used in the German-speaking part of Switzerland as part of their “risk-oriented criminal justice” . The tool groups incarcerated people into three risk categories. A high risk leads to further screening by specialists. It is known which data points the tool uses for this assessment (e.g., previous convictions or age), but not how the risk category is calculated from these data. Thus, it is unclear whether the risk assessment works equally well for all groups or whether there are groups that are systematically disadvantaged by the tool.
In the U.S. healthcare system, a commercial tool is used to decide which patients will have access to a care program with additional and more personalized care. The idea is to make this decision on the basis of individual need. An algorithm estimates need based on available data from the health care system, in particular health care costs: Higher costs are assumed to indicate higher need. A study has now shown that the costs for Black patients are on average lower than for white patients with comparable health conditions. As a result, their need for personalized care is perceived to be lower and they are less likely to be enrolled in the care program.
A scientific study was able to demonstrate gender differences in job ads on Facebook for technical careers: An ad that the researchers distributed on Facebook worldwide was shown substantially more often to men than to women, even though women were more likely to respond. The suspected reason is Facebook's business model, particularly the ad pricing. A similar difference in exposure to job ads between women and men has also been demonstrated on other platforms such as Google, Instagram, and Twitter.
In a study of commercial facial recognition systems, it was shown that the facial recognition systems of Microsoft, IBM and Face++ are significantly less accurate for women with “dark” skin than for men with “light” skin. For women, the error rate, i.e., the percentage of incorrectly recognized faces, ranged from 10.7 to 21.3%, while for men it ranged from 0.7 to 5.6%. The error rate for people with “dark” skin ranged from 12.9 to 22.4 %, and for people with “light” skin from 0.7 to 4.7 %. Particularly striking is the error rate for women with “dark” skin: between 20.8 and 34.7 %. For men with “light” skin, this rate was 0.0 to 0.8 %.
Predictive policing analyzes large amounts of crime data to identify patterns and predict future crimes. These predictions are used to decide where police officers should be deployed. If neighborhoods, where marginalized groups live, are policed particularly heavily, more crimes will be detected there — even if the crime rate is actually no higher than elsewhere. A tool that works with this biased data will predict more crimes in these areas. As a result, more police officers will be deployed there, leading to a self-reinforcing effect.
In 2020, A-level exams could not take place in the United Kingdom due to the COVID-19 pandemic. Grades were determined using an algorithm. This algorithm used teachers' assessments, a ranking of students, and grades from previous years at that school. Nearly 40% of all students received a lower grade than their teachers' assessment. Especially students from private schools benefited from the algorithm. Thus, students from wealthier families were more likely to benefit from the algorithm than those from poorer families. The impact on students' lives is significant as grades determine which universities students can attend.
Munich-based startup Retorio has developed a tool that creates personality profiles based on video interviews. Instead of conducting personal interviews, job applicants can be pre-selected using this video interview tool, which saves time. For this reason, such tools are now widely used. However, experiments conducted by Bayerischer Rundfunk (BR) with Retorio's tool have called its reliability into question: If a person wears glasses, they are evaluated differently than without. A bookshelf in the background or an adjustment of the video's brightness changes the results as well. Thus, it is unclear whether the tool provides any meaningful results at all.
The Austrian employment agency is developing a machine learning tool to predict how employable unemployed people are. The tool is intended to help distribute the office's resources more efficiently. Those who have very good prospects of finding a job receive less support as this does not add any value. Those who have very poor prospects of finding a job also receive less support. Those who have worked little in the previous year are considered to be less employable — the prospect of support decreases. A health impairment, having given birth or being a woman also lowers the estimated employability. This has led to the tool being heavily criticized as it systematically disadvantages certain groups.
Automating Society Report, https://automatingsociety.algo...
Research project about socially acceptable AI, https://fair-ai.ch/
Automated decision making systems in the public administration, https://algorithmwatch.ch/de/a...
Facebook campaign, https://algorithmwatch.org/de/...
European Workshop on Algorithmic Fairness (EWAF) in Zurich, https://sites.google.com/view/...
Coded Bias (film, on Netflix)
Eubanks, Virginia: Automating Inequality: How High-Tech Tools Profile, Police, and Punish the Poor. St. Martin's Press, 2018. (book, ISBN 9781250074317).
O'Neil, Cathy: Weapons of Math Destruction. How Big Data Increases Inequality and Threatens Democracy. Crown, 2016 (book, ISBN 0553418815)
Benjamin, Ruha: Race After Technology: Abolitionist Tools for the New Jim Code. Polity, 2019 (book, ISBN 9781509526390).
Concept: Corinna Hertweck, School of Engineering, ZHAW
Project management and scientific support: Prof. Dr. Christoph Heitz, School of Engineering, ZHAW
Concept: Tobias Urech, AlgorithmWatch Switzerland
Project management and expert support: Dr. Anna Mätzener, AlgorithmWatch Switzerland
Collaboration: Tristesse, Basel